Boosting Sales with AI Recommendation Systems
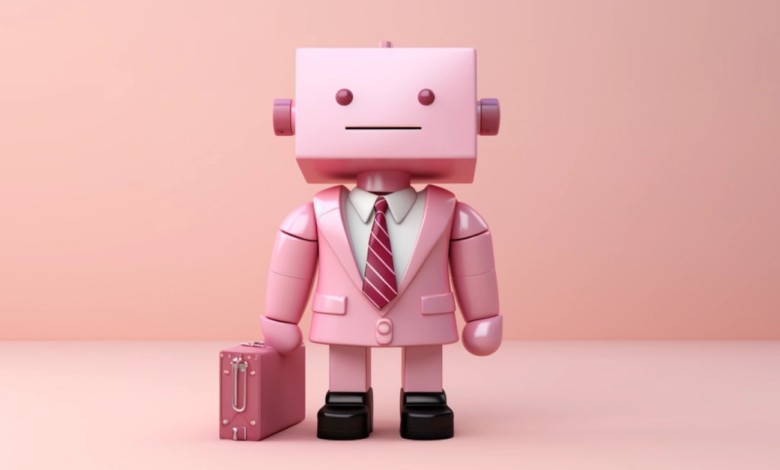
Unlock the Power of AI Recommendation Systems for Your Ecommerce Store
Is there anything more powerful than a word-of-mouth recommendation? When a trusted friend suggests a new book or skin care brand, you know they’ve got your best interest at heart—and understand you better than just about anyone.
But what if you had a friend with nearly infinite information about the things you love? Their recommendations would be laser-focused, perfectly matching your needs and desires. That’s the promise of an AI recommendation system.
Ecommerce stores can leverage artificial intelligence to analyze user data and deliver more relevant recommendations. For many businesses, an AI recommendation system may be the boost your sales and marketing teams need—and it’s all automated.
What is an AI recommendation system?
An AI recommendation system—also known as a recommendation engine—uses machine learning algorithms to suggest relevant products, services, or content to potential customers online.
AI recommendation systems collect and analyze data points like demographics, a user’s past behavior (reviews, ratings, search history, past purchases) and product attributes, creating personalized recommendations.
A wide range of industries—entertainment, finance, marketing, and ecommerce—use AI-powered recommendation engines. For example, an ecommerce merchant might rely on an AI recommendation system to display data-driven suggestions to site visitors. Gymshark’s recommendation engine, for instance, recommends products based on customers with similar interests.
How an AI recommendation system can increase your sales
A good AI-powered recommendation system can personalize your online store experience, driving repeat purchases and higher satisfaction. Nearly half of US shoppers in 2023 wanted personalized product recommendations, and 56% of customers reported returning to a merchant after a customized shopping experience. By personalizing the shopping experience, AI recommendation systems can also increase customer retention and improve the overall customer experience.
Key benefits include:
- Streamlined discovery. The system surfaces highly relevant choices, so customers don’t have to scroll endlessly to find items they want.
- Higher average order value. “Customers also bought” or “Frequently bought together” sections on your checkout page can encourage upsells and cross-sells, increasing average order value and boosting revenue.
- Enhanced customer loyalty. A faster, more personalized experience as a result of an AI recommendations system keeps shoppers coming back.
Types of AI recommendation systems
You can generate personalized recommendations for potential customers using one of three main types of AI recommendation systems:
Content-based filtering
An AI recommendation system using content-based filtering algorithms makes recommendations based on the specific characteristics (features, categories, descriptions) of items a user already likes, rather than what other users do. By analyzing product metadata using data filtering, an AI recommendation system can recommend items with similar attributes to those a shopper has previously engaged with.
This works particularly well for niche markets with a limited customer base. You may not have a lot of customer reviews or interactions on your site, but if you have sufficient product information, you can use a content-based filtering system to make recommendations instead.
For example, the Shopcast app uses content-based filtering to make recommendations based on similar product features.
Collaborative filtering
Collaborative filtering predicts a user’s preferences based on the behavior of similar users. Collaborative filtering systems may consider browsing history, purchase history, or ratings. This type of recommendation engine suggests products it thinks you’ll like based on customer data from shoppers with similar tendencies. For example, a clothing brand might recommend a new clothing line to a particular user based on recent fashion purchases of users with similar tastes.
As opposed to content-based filtering, collaborative filtering is useful for suggesting items not directly related to products the user has viewed but that similar customers have purchased. For example, Also Bought is an AI-powered recommendation system that recommends products that are commonly purchased alongside items in a customer’s cart.
Hybrid filtering
Hybrid recommendation systems combine collaborative and content-based filtering to recommend relevant content, products, and services to users. For example, hybrid systems could predict the type of cookware that might interest a customer based on what other users with similar preferences have chosen, and specific content-based connections in product features, materials, or sizes.
By collecting data about similarities between users and content-based factors like product descriptions, a hybrid recommendation system combines multiple filtering methods to get a highly informed and personalized recommendation.
Popular AI recommendation engines typically rely on a hybrid approach, applying both collaborative and content-based filtering. For example, the FT: Frequently Bought Together tool creates automatic product recommendations based on user behavior and real-time order data.
AI recommendation system FAQ
What is an example of an AI recommendation system?
The FT: Frequently Bought Together app is an example of an AI-powered recommendation system that uses collaborative filters to recommend products to your customers based on data like user preferences and user engagement.
What’s a disadvantage of recommendation systems?
A disadvantage of AI recommendation systems is the large amount of user data gathered, which can result in privacy concerns.
How are AI recommendation systems used in ecommerce?
Ecommerce merchants use AI recommendation systems to generate personalized recommendations for products or services based on user behavior and product attributes.
What are the benefits of using AI recommendation systems in ecommerce?
AI recommendation systems in ecommerce can streamline product discovery, increase average order value, enhance customer loyalty, and improve overall customer experience.
How can AI recommendation systems help increase sales and revenue?
By providing personalized recommendations to customers, AI recommendation systems can drive repeat purchases, encourage upsells and cross-sells, and ultimately boost sales and revenue for ecommerce businesses.
following sentence:
“The cat sat lazily in the sun, enjoying the warmth on its fur.”
The cat lounged in the sunlight, basking in the cozy heat against its fur.